In the ever-changing healthcare sector, integrating advanced technologies is critical to effectively managing complex systems. Generative AI is one of the most promising advances in this industry, since it transforms numerous aspects of the healthcare revenue cycle. This paper looks at how Generative AI is transforming hospital revenue management, increasing accuracy, and improving operational efficiency.
Understanding Generative AI
Generative AI refers to artificial intelligence technology that can generate new material, data, or insights from previously collected information. Unlike classical AI, which may classify or predict outcomes based on pre-established patterns, generative AI generates fresh and contextually relevant data. In the context of healthcare, this technology has the potential to greatly improve the revenue cycle by reducing operations and increasing accuracy.
The Healthcare Revenue Cycle: A Brief Overview
The healthcare revenue cycle consists of several crucial stages:
Patient Scheduling and Registration: Obtaining and verifying patient information.
Insurance Verification: Ensuring that patient insurance information is correct, and coverage is validated.
Charge Capture: Documenting services rendered and converting them into billable costs.
Claim Submission: Filing claims with insurance companies for compensation.
Payment Collection: Managing patient and insurance payments, resolving denials, and assuring proper posting.
Each of these stages entails complex processes that necessitate precision and efficiency to ensure that healthcare practitioners are fairly compensated for their services.
How Generative AI is Revolutionizing the Healthcare Revenue Cycle
Improved Accuracy in Claim Submission
One of the key advantages of incorporating Generative AI into the healthcare revenue cycle is its capacity to improve claim accuracy. Traditional techniques frequently need manual entry and substantial paperwork, which can lead to mistakes and delays. Generative AI can automate and streamline these processes by creating precise claims based on extensive data analysis. This reduces human mistake and enhances the possibility that insurance companies will accept first-pass claims.
Improved Patient Data Management
The healthcare revenue cycle relies heavily on excellent patient data management. Generative AI can help organize and analyse vast amounts of patient data, ensuring that all essential information is captured and used properly. For example, AI systems can cross-reference patient data with insurance information to detect anomalies or missing information before claims are made. This lowers the chance of claim denials and speeds up the reimbursement procedure.
Streamlined Charge Capture
Charge capture entails documenting all services rendered and converting them into chargeable charges. Generative AI can help with this process by automatically creating proper charge codes based on the services reported in electronic health records (EHRs). This not only speeds up the charge capture process, but also decreases the possibility of coding errors, ensuring that providers are properly reimbursed for the services they provide.
Automated Denial Management
Denial management is an important part of the healthcare revenue cycle. When claims are denied, healthcare providers must determine the reasons for the denial and resolve the concerns before resubmitting the claims. Generative AI can study rejection patterns and develop insights about prevalent problems, allowing providers to solve them pre-emptively. AI-powered solutions can help automate the resubmission of erroneous claims, reducing administrative stress on staff and increasing overall efficiency.
Predictive Analytics in Financial Planning
Generative AI can use predictive analytics to estimate revenue and improve financial planning. By examining historical data and recognizing trends, AI systems can forecast future revenue streams and financial issues. This allows healthcare businesses to make more informed decisions about resource allocation, budget planning, and strategic investments.
The Future of Generative AI in Healthcare
The future of generative AI in healthcare is poised to revolutionize patient care, medical research, and operational efficiency. By 2030, the global AI in healthcare market is expected to reach $194.4 billion, with generative AI playing a significant role in areas such as drug discovery, personalized medicine, and diagnostic support. For example, AI-driven models can generate new drug compounds in a fraction of the time it takes traditional methods, potentially reducing the drug development timeline by 70%. Additionally, the ability to simulate complex biological processes using AI could lead to more accurate disease models, improving the effectiveness of treatments and accelerating clinical trials.
Generative AI is also transforming how healthcare professionals approach diagnostics and patient care. With its ability to analyze vast datasets and generate predictive models, AI is enhancing early detection of diseases like cancer and neurological disorders. The integration of generative AI in SaaS development is further driving innovation, allowing healthcare providers to access advanced diagnostic tools and personalized treatment plans through scalable, cloud-based platforms. By 2025, AI-powered diagnostic tools could reduce diagnostic errors by up to 85%, leading to more precise and timely interventions. Moreover, generative AI is expected to support the creation of personalized treatment plans by generating tailored therapeutic approaches based on a patient’s genetic makeup, lifestyle, and medical history, ushering in a new era of precision medicine.
How AI Helps in Healthcare Beyond Revenue Cycle Management
Generative AI’s uses extend beyond revenue cycle management to numerous other elements of healthcare:
Predictive Analytics for Disease Prevention:
Patient data is analyzed to predict the likelihood of diseases like diabetes, heart conditions, and cancer. Early detection enables preventive measures and personalized treatment plans, leading to improved patient outcomes.
Medical Imaging and Diagnostics:
Accuracy in interpreting medical images, such as X-rays, MRIs, and CT scans, is significantly enhanced. Anomalies that might be missed by the human eye are identified, leading to earlier and more accurate diagnoses.
Drug Discovery and Development:
The process of drug discovery is accelerated by predicting interactions between compounds and biological targets. This reduces the time and cost of bringing new drugs to market and aids in repurposing existing drugs.
Personalized Treatment Plans:
Genetic, lifestyle, and clinical data are analyzed to create personalized treatment plans. Patients receive the most effective therapies based on their unique profiles, improving treatment success rates.
Virtual Health Assistants:
Patients receive round-the-clock support through virtual assistants, which answer health-related questions, remind them of medications, and help manage chronic conditions, enhancing patient engagement and adherence.
Robotic Surgery:
Surgeons are assisted with precision and control during complex procedures. Robotic systems guided by advanced algorithms perform minimally invasive surgeries, reducing risk and speeding up recovery times.
Remote Patient Monitoring:
Continuous monitoring of patients with chronic conditions is enabled through wearable devices and sensors. Healthcare providers receive alerts for significant changes in health status, allowing timely interventions.
Operational Efficiency and Workflow Optimization:
Hospital operations, such as patient scheduling, bed management, and resource allocation, are optimized. This reduces wait times, improves staff utilization, and enhances overall efficiency in healthcare delivery.
AI Applications in Healthcare Revenue Cycle Management
Here are AI applications in Healthcare Revenue Cycle Management (RCM)
Automated Coding and Billing:
AI automates coding and billing, reducing manual errors and increasing efficiency. This speeds up claim submissions, minimizes rework, and ensures accurate reimbursement, enhancing the overall financial health of healthcare providers.
Revenue Integrity:
AI continuously monitors documentation, coding, and billing for compliance, ensuring accurate revenue capture. It helps detect discrepancies early, maintaining financial integrity and maximizing reimbursements.
Patient Eligibility Verification:
AI verifies patient insurance coverage in real-time, preventing claim denials due to eligibility issues. This accelerates the claims process and reduces administrative burdens, leading to faster payments.
Fraud Detection:
AI detects unusual billing patterns, helping to identify and prevent fraudulent activities. This protects healthcare providers from financial losses and regulatory penalties.
Prior Authorization Automation:
AI automates the prior authorization process, quickly gathering required documentation and reducing delays. This improves patient care timelines and reduces administrative overhead.
Patient Communication and Engagement:
AI-driven chatbots and virtual assistants handle billing inquiries, payment reminders, and support, enhancing patient engagement. This leads to better patient satisfaction and timely revenue collection.
Conclusion
Generative AI will change healthcare by making drug discovery faster, improving diagnostics, and creating personalized treatments. As these technologies grow, patient care will become more precise and effective, benefiting both doctors and patients.
Explore these innovations firsthand and see how AI can transform your healthcare solutions. For a free trial, register today at www.ezinsights.ai.
FAQs
How does generative AI improve the efficiency of the healthcare revenue cycle?
Generative AI improves the healthcare revenue cycle by automating repetitive and time-consuming operations like claims processing, coding, and billing. AI systems can swiftly evaluate enormous amounts of data, find problems, and recommend corrections, considerably accelerating these operations. This decreases the administrative burden on workers, shortens delays, and increases income flow.
In what ways does generative AI contribute to the accuracy of medical coding and billing?
Generative AI increases medical coding and billing accuracy by using complex algorithms to assess patient records, clinical notes, and coding standards. AI systems can cross-reference these inputs to guarantee that codes and billing information are accurate and in line with current standards. This lowers the likelihood of errors, denials, and underpayments, resulting in more accurate and compliant claims.
What are the potential challenges of implementing generative AI in the healthcare revenue cycle?
Implementing generative AI in the healthcare revenue cycle presents challenges such as data privacy concerns, the necessity for solid interaction with existing systems, and the possibility of opposition from staff accustomed to old practices. Furthermore, maintaining the AI system’s correctness and reliability necessitates ongoing training and updates to accommodate changing coding requirements and laws.
How can healthcare organizations measure the impact of generative AI on their revenue cycle operations?
Healthcare firms can assess the impact of generative AI by analyzing key performance measures such as claim denial rates, claim processing times, coding accuracy, and overall revenue cycle efficiency. A comparative comparison of these measures before and after AI installation can reveal gains in efficiency, accuracy, and financial results.
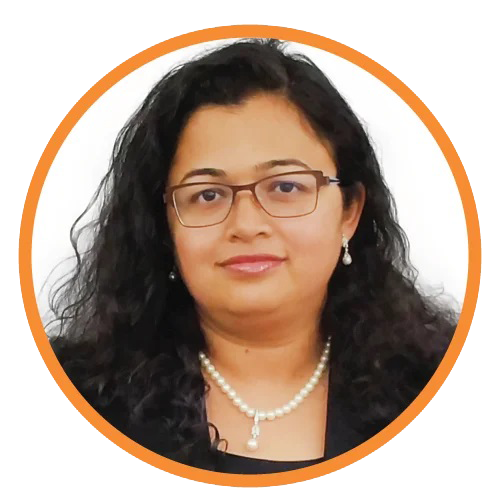
Anupama Desai
President & CEO
Anupama has more than 23 years of experience as business leader and as an advocate for improving the life of the business users. Anupama has been very active in bringing business perspective in the technology enabled world. Her passion is to leverage information and data insights for better business performance by empowering people within the organization. Currently, Anupama leads Winnovation to build world class Business Intelligence application platform and her aim is to provide data insights to each and every person within an organization at lowest possible cost.