Data is king in today’s fast-paced digital world. Effective data collection and analysis skills may make or break companies in a variety of sectors. The amount of data being generated is growing at an exponential rate, making traditional analytics techniques inadequate. Here’s where augmented analytics comes into play, completely changing how businesses use data to gain insights. We’ll examine the idea of augmented analytics in this extensive tutorial, along with a step-by-step plan for implementing this game-changing technology.
Understanding Augmented Analytics
Fundamentally, augmented analytics improves data preparation, analysis, and interpretation by fusing machine learning, natural language processing, and AI-driven automation. Augmented analytics enables users of all skill levels to find insightful information, in contrast to standard analytics approaches that call for manual intervention and knowledge. Here are some crucial steps to keep in mind with regards to Augmented Analytics: –
Step 1: Grasping the Basics
It’s important to understand the basics before getting into augmented analytics. Learn the terms for important concepts including predictive modeling, machine learning algorithms, and data visualization methods. Gaining an understanding of these ideas will set you up for success as you explore augmented analytics.
Step 2: Exploring Augmented Analytics Platforms
Investigate augmented analytics tools that are suited to your business requirements and industry as the next step. These systems use cutting-edge technologies to instantly produce actionable insights, recognize patterns in data, and automate data preparation. There are numerous augmented analytics tools available to help you optimize your data analysis procedures, regardless of your industry—healthcare, banking, or retail.
Step 3: Using Augmented Analytics in Healthcare
Augmented analytics has enormous potential to improve patient outcomes and operational efficiency in the healthcare sector. Augmented analytics can help healthcare providers diagnose ailments, spot patterns, and allocate resources more efficiently. It can do this through predictive modeling and disease surveillance, among other things. Healthcare companies may provide more individualized care and promote innovation in medical research by utilizing augmented analytics.
Step 4: Embracing Augmented Reality Analytics
Users can view and interact with data in immersive environments thanks to augmented reality analytics, which is the confluence of augmented reality (AR) with data analytics. This creative method applies ideas to the real world to improve decision-making and data investigation. Augmented reality analytics adds a new level to data analysis, whether it is used to simulate patient outcomes in a healthcare environment or visualize sales performance in a retail store.
Step 5: Integrating Augmented Analytics into Your Workflow
It’s time to include augmented analytics principles and platforms into your workflow now that you are familiar with them. Determine use cases first, where augmented analytics can lead to concrete business results. Work together with data scientists and business analysts to create tailored solutions that meet the unique goals and difficulties faced by your company.
Step 6: Continuous Learning and Optimization
The process of adopting augmented analytics is ongoing even after a solution has been put into place. Make a commitment to ongoing optimization and learning to stay ahead of the curve. Stay up to date with the latest advancements, innovations, and best practices in the augmented analytics area. Utilize performance measurements and feedback loops to improve your analytics tactics and guarantee continued success.
What Are Augmented Analytics Platforms?
Augmented analytics platforms integrate advanced technologies such as AI and ML to automate various stages of the analytics workflow. This includes data preparation, insight generation, and even the interpretation of results. By automating these processes, augmented analytics platforms reduce the burden on data scientists and make analytics accessible to non-technical users. This democratization of data empowers a wider range of employees to engage with data, uncover patterns, and make informed decisions.
Benefits of Using Augmented Analytics Platforms
Enhanced Efficiency:
Automation of data preparation and analysis significantly reduces the time and effort required to generate insights, allowing organizations to respond quickly to changing conditions.
Accessibility for All Users:
By lowering the technical barriers, augmented analytics platforms enable a broader range of employees to engage with data, fostering a data-driven culture across the organization.
Improved Accuracy:
AI and ML algorithms can process vast amounts of data with high precision, minimizing the risk of human error and enhancing the reliability of insights.
Informed Decision Making:
With AI-generated insights and predictive analytics, organizations can make more informed decisions, anticipate future trends, and proactively address challenges.
7 Key Trends in Augmented Analytics
The field of augmented analytics is rapidly evolving, driven by advancements in artificial intelligence (AI) and machine learning (ML). As organizations increasingly recognize the value of data-driven decision-making, several key trends are shaping the future of augmented analytics. Here are seven trends to watch:
Integration of Natural Language Processing (NLP)
Natural Language Processing (NLP) is revolutionizing how users interact with data. Augmented analytics platforms now enable users to query data using everyday language, making complex data analysis accessible to non-technical users. This trend is enhancing user experience and fostering a more intuitive approach to data exploration.
Enhanced Data Quality Management
Data quality is paramount for accurate insights. Augmented analytics platforms are incorporating advanced data quality management features, such as automated data cleansing, anomaly detection, and data enrichment. These enhancements ensure that the data being analyzed is accurate, complete, and reliable, leading to more trustworthy insights.
Automated Data Preparation
Manual data preparation can be a time-consuming and error-prone process. Augmented analytics platforms are increasingly automating data preparation tasks, including data integration, cleaning, and transformation. This automation reduces the burden on data scientists and speeds up the analytics process, allowing organizations to derive insights faster.
Advanced Predictive and Prescriptive Analytics
Predictive analytics forecasts future trends based on historical data, while prescriptive analytics recommends actions to achieve desired outcomes. Augmented analytics platforms are enhancing these capabilities, providing more accurate and actionable insights. Organizations can now anticipate future scenarios and make proactive decisions to optimize their operations.
Increased Adoption of AI and ML Models
The adoption of AI and ML models in augmented analytics is accelerating. These models are becoming more sophisticated, enabling deeper and more complex data analyses. As a result, businesses can uncover hidden patterns, correlations, and insights that were previously unattainable with traditional analytics methods.
Focus on Explainable AI
As AI-driven insights become more prevalent, the need for explainable AI (XAI) is growing. Users must understand how AI models arrive at their conclusions to trust and act on the insights. Augmented analytics platforms are integrating XAI features, providing transparent and interpretable explanations of AI-generated insights.
Personalized User Experiences
Personalization is key to user engagement and satisfaction. Augmented analytics platforms are leveraging user behavior data to deliver personalized experiences, such as tailored dashboards, reports, and recommendations. This trend ensures that users receive relevant and actionable insights that align with their specific roles and needs.
Implementing Augmented Analytics Platforms
Implementing augmented analytics platforms involves several key steps:
Data Integration and Preparation:
Start by integrating and cleansing data from multiple sources to ensure accuracy and consistency.
Model Building and Training:
Develop and train machine learning models tailored to specific business needs, such as anomaly detection or customer segmentation.
Deployment and Integration:
Integrate the models with existing analytics platforms and business applications and deploy them into operational systems.
User Adoption and Training:
Educate users on how to interpret and apply the insights generated by the platform, ensuring they can leverage the technology effectively.
Continuous Improvement: Regularly update models and processes based on user feedback and evolving business requirements to maintain optimal performance.
Conclusion
To sum up, augmented analytics is a paradigm shift in the way we interpret data and draw conclusions from it. Organizations may achieve new heights of productivity, innovation, and competitiveness by combining the power of machine learning, automation, and AI-driven algorithms. In the data-driven era, adopting augmented analytics is essential to remain ahead of the curve, whether you work in banking, healthcare, or any other industry. To maximize the potential of your data assets and start your road towards augmented analytics excellence, adhere to this step-by-step guidance.
FAQs
What exactly is augmented analytics?
Augmented analytics uses AI and machine learning to enhance data analytics by automating data preparation, analysis, and insight generation, empowering both technical and non-technical users to make data-driven decisions.
How does augmented analytics differ from traditional analytics?
Unlike traditional analytics that rely on manual efforts by data scientists, augmented analytics employs AI and automation to streamline the entire analytics process, democratizing access to insights across the organization.
What are the key steps in implementing augmented analytics?
Implementing augmented analytics involves integrating and cleansing data, building and training machine learning models, deploying models into systems, training users, and continuously updating models for optimal performance.
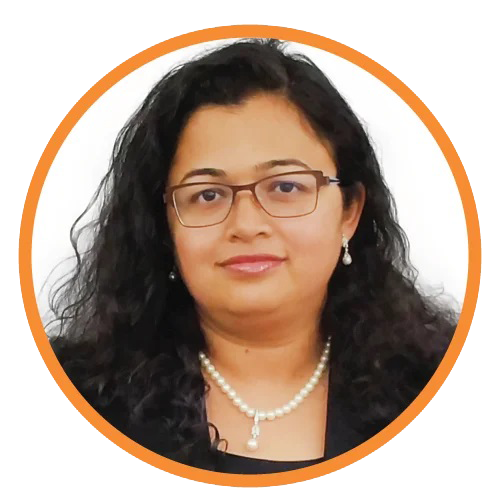
Anupama Desai
President & CEO
Anupama has more than 23 years of experience as business leader and as an advocate for improving the life of the business users. Anupama has been very active in bringing business perspective in the technology enabled world. Her passion is to leverage information and data insights for better business performance by empowering people within the organization. Currently, Anupama leads Winnovation to build world class Business Intelligence application platform and her aim is to provide data insights to each and every person within an organization at lowest possible cost.